Biased Artificial Intelligence (AI)
Biases find their way into the designed AI systems, and are used to
make decisions by many, from governments to businesses. Bad data
used to train AI can contain implicit racial, gender, or ideological
biases. Bias in AI systems could erode trust between humans and
machines that learn.
Untold History of AI: Algorithmic Bias Was
Born in the 1980s
The history of AI is
often told as the story of machines getting smarter over time.
What’s lost is the human element in the narrative, how intelligent
machines are designed, trained, and powered by human minds and
bodies.
In this six-part
series, we explore that human history of AI—how innovators,
thinkers, workers, and sometimes hucksters have created algorithms
that can replicate human thought and behavior (or at least appear
to). While it can be exciting to be swept up by the idea of
superintelligent computers that have no need for human input, the
true history of smart machines shows that our AI is only as good as
we are.
Part 5: Franglen’s
Admissions Algorithm
In the 1970s, Dr. Geoffrey Franglen of St. George’s Hospital Medical
School in London began writing an algorithm to screen student
applications for admission.
At the time,
three-quarters of St. George’s 2,500 annual applicants were rejected
by the academic assessors based on their written applications alone,
and didn’t get to the interview stage. About 70 percent of those who
did make it past the initial screening went on to get offered places
at the medical school. So that initial “weeding out” round was
crucial.
Franglen was the
vice-dean of St. George’s and himself an admissions assessor.
Reading through the applications was a time-consuming task that he
felt could be automated. He studied the processes by which he and
his colleagues screened students, then wrote a program that, in his
words, “mimic[ked] the behavior of the human assessors.”
While Franglen’s
main motivation was to make admissions processes more efficient, he
also hoped that it would remove inconsistencies in the way the
admissions staff carried out their duties. The idea was that by
ceding agency to a technical system, all student applicants would be
subject to precisely the same evaluation, thus creating a fairer
process.
In fact, it proved
to be just the opposite.
Franglen completed
the algorithm in 1979. That year, student applicants were
double-tested by the computer and human assessors. Franglen found
that his system agreed with the gradings of the selection panel 90
to 95 percent of the time. For the medical school’s administrators,
this result proved that an algorithm could replace the human
selectors. By 1982, all initial applications to St. George’s were
being screened by the program.
If their names were
non-Caucasian, the selection process was weighted against them. In
fact, simply having a non-European name could automatically take 15
points off an applicant’s score.
Within a few years,
some staff members had become concerned by the lack of diversity
among successful applicants. They conducted an internal review of
Franglen’s program and noticed certain rules in the system that
weighed applicants on the basis of seemingly non-relevant factors,
like place of birth and name. But Franglen assured the committee
that these rules were derived from data collected from previous
admission trends and would have only marginal impacts on selections.
In December 1986,
two senior lecturers at St. George’s caught wind of this internal
review and went to the U.K. Commission for Racial Equality. They had
reason to believe, they informed the commissioners, that the
computer program was being used to covertly discriminate against
women and people of color.
The commission
launched an inquiry. It found that candidates were classified by the
algorithm as “Caucasian” or “non-Caucasian” on the basis of their
names and places of birth. If their names were non-Caucasian, the
selection process was weighted against them. In fact, simply having
a non-European name could automatically take 15 points off an
applicant’s score. The commission also found that female applicants
were docked three points, on average. As many as 60 applications
each year may have been denied interviews on the basis of this
scoring system.
At the time, gender
and racial discrimination was rampant in British universities—St.
George’s was only caught out because it had enshrined its biases in
a computer program. Because the algorithm was verifiably designed to
give lower scores to women and people with non-European names, the
commission had concrete evidence of discrimination.
St. George’s was
found guilty by the commission of practicing discrimination in its
admissions policy, but escaped without significant consequences. In
a mild attempt at reparations, the college tried to contact people
who might have been unfairly discriminated against, and three
previously rejected applicants were offered places at the school.
The commission noted
that the problem at the medical school was not only technical, but
also cultural. Many staff members viewed the admissions machine as
unquestionable, and therefore didn’t take the time to ask how it
distinguished between students.
At a deeper level,
the algorithm was sustaining the biases that already existed in the
admissions system. After all, Franglen had tested the machine
against humans and found a 90 to 95 percent correlation of outcomes.
But by codifying the human selectors’ discriminatory practices into
a technical system, he was ensuring that these biases would be
replayed in perpetuity.
The case of
discrimination at St. George’s got a good deal of attention. In the
fallout, the commission mandated the inclusion of race and ethnicity
information in university admission forms. But that modest step did
nothing to stop the insidious spread of algorithmic bias.
Indeed, as
algorithmic decision-making systems are increasingly rolled out into
high-stakes domains, like health care and criminal justice, the
perpetuation and amplification of existing social biases based on
historical data has become an enormous concern. In 2016, the
investigative journalism outfit ProPublica showed that software used
across the United States to predict future criminals was biased
against African-Americans. More recently, researcher Joy Buolamwini
demonstrated that Amazon’s facial recognition software has a far
higher error rate for dark-skinned women.
While machine bias
is fast becoming one of the most discussed topics in AI, algorithms
are still often perceived as inscrutable and unquestionable objects
of mathematics that produce rational, unbiased outcomes. As AI
critic Kate Crawford says, it’s time to recognize that algorithms
are a “creation of human design” that inherit our biases. The
cultural myth of the unquestionable algorithm often works to conceal
this fact: Our AI is only as good as we are (Untold
History of AI: Algorithmic Bias Was Born in the 1980s - IEEE
Spectrum).
Untold History of AI: When Charles Babbage Played Chess With the
Original Mechanical Turk - IEEE Spectrum (Part 1)
Untold History of AI: Invisible Women Programmed America's First
Electronic Computer - IEEE Spectrum (Part 2)
Untold History of AI: Why Alan Turing Wanted AI Agents to Make
Mistakes - IEEE Spectrum (Part 3)
Untold History of AI: The DARPA Dreamer Who Aimed for Cyborg
Intelligence - IEEE Spectrum (Part 4)
Untold History of AI: Algorithmic Bias Was Born in the 1980s - IEEE
Spectrum (Part 5)
Untold History of AI: How Amazon’s Mechanical Turkers Got Squeezed
Inside the Machine - IEEE Spectrum (Part 6)
Ontology
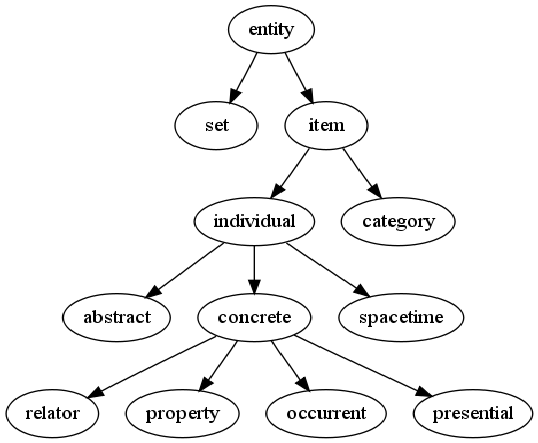
An ontology
represents knowledge as a set of concepts within a domain and the
relationships between those concepts.
1. the branch of metaphysics dealing with the nature of being.
2. a set of concepts and categories in a subject area or domain that
shows their properties and the relations between them.
"what's new about
our ontology is that it is created automatically from large
datasets"
Knowledge
representation
Knowledge
representation and knowledge engineering are central to classical AI
research. Some "expert systems" attempt to gather together explicit
knowledge possessed by experts in some narrow domain. In addition,
some projects attempt to gather the "commonsense knowledge" known to
the average person into a database containing extensive knowledge
about the world. Among the things a comprehensive commonsense
knowledge base would contain are: objects, properties, categories
and relations between objects; situations, events, states and time;
causes and effects; knowledge about knowledge (what we know about
what other people know); and many other, less well researched
domains.
A representation of
"what exists" is an ontology: the set of objects, relations,
concepts, and properties formally described so that software agents
can interpret them. The semantics of these are captured as
description logic concepts, roles, and individuals, and typically
implemented as classes, properties, and individuals in the Web
Ontology Language.
The most general
ontologies are called upper ontologies, which attempt to provide a
foundation for all other knowledge by acting as mediators between
domain ontologies that cover specific knowledge about a particular
knowledge domain (field of interest or area of concern). Such formal
knowledge representations can be used in content-based indexing and
retrieval, scene interpretation, clinical decision support,
knowledge discovery (mining "interesting" and actionable inferences
from large databases), and other areas.
Among the most difficult problems in knowledge representation are:
-Default reasoning
and the qualification problem
-Breadth of commonsense knowledge
-Subsymbolic form of some commonsense knowledge (Wikipedia).
About TACS
TACS
Consulting Delivers The Insight and Vision on Information
Communication and Energy Technologies for Strategic Decisions.
TACS is Pioneer and Innovator of many Communication Signal
Processors, Optical Modems, Optimum or Robust Multi-User or
Single-User MIMO Packet Radio Modems, 1G Modems, 2G Modems, 3G
Modems, 4G Modems, 5G Modems, 6G Modems, Satellite Modems, PSTN
Modems, Cable Modems, PLC Modems, IoT Modems and more..
TACS consultants conducted fundamental scientific research in
the field of communications and are the pioneer and first
inventors of PLC MODEMS, Optimum or Robust Multi-User or
Single-User MIMO fixed or mobile packet radio structures in the
world.
TACS is a leading top consultancy in the field of Information,
Communication and Energy Technologies (ICET). The heart of our
Consulting spectrum comprises strategic, organizational, and
technology-intensive tasks that arise from the use of new
information and telecommunications technologies. TACS Consulting
offers Strategic Planning, Information, Communications and
Energy Technology Standards and Architecture Assessment, Systems
Engineering, Planning, and Resource Optimization.
|
TACS is a leading top consultancy in the field of information, communication
and energy technologies (ICET).
The heart of our consulting spectrum comprises strategic,
organizational, and technology-intensive tasks that arise from the use of new
information, communication and energy technologies. The major emphasis in our work is found in innovative consulting and
implementation solutions which result from the use of modern information,
communication and energy technologies.
TACS
- Delivers the insight and vision
on technology for strategic decisions
- Drives
innovations forward as part of our service offerings to customers
worldwide
- Conceives
integral solutions on the basis of our integrated business and technological
competence in the ICET sector
- Assesses technologies and standards and develops
architectures for fixed or mobile, wired or wireless communications systems
and networks
- Provides
the energy and experience of world-wide leading innovators and experts in their fields for local,
national or large-scale international projects.
|